The Sobering Truth About the Impact of Your Business Ideas
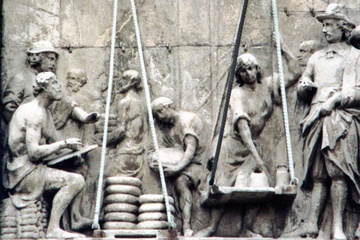
The introduction of data science into the business world has contributed far more than recommendation algorithms; it has also taught us a lot about the efficacy with which we manage our businesses. Specifically, data science has introduced rigorous methods for measuring the outcomes of business ideas. These are the strategic ideas that we implement in order to achieve our business goals. For example, “We’ll lower prices to increase demand by 10%” and “we’ll implement a loyalty program to improve retention by 5%.” Many companies simply execute on their business ideas without measuring if they delivered the impact that was expected. But, science-based organizations are rigorously quantifying this impact and have learned some sobering lessons:
- The vast majority of business ideas fail to generate a positive impact.
- Most companies are unaware of this.
- It is unlikely that companies will increase the success rate for their business ideas.
These are lessons that could profoundly change how businesses operate. In what follows, we flesh out the three assertions above with the bulk of the content explaining why it may be difficult to improve the poor success rate for business ideas. Despite the challenges, we conclude with some recommendations for better managing your business.
(1) The vast majority of business ideas fail to generate positive results
To properly measure the outcomes of business ideas, companies are embracing experimentation (a.k.a. randomized controlled trials or A/B testing). The process is simple in concept. Before rolling out a business idea, you test; you try the idea out on a subset group of customers1 while another group—a control group—is not exposed to the new idea. When properly sampled, the two groups will exhibit the same attributes (demographics, geographics, etc.) and behaviors (purchase rates, life-time-value, etc.). Therefore, when the intervention is introduced—ie. the exposure to the new business idea—any changes in behavior can be causally attributed to the new business idea. This is the gold standard in scientific measurement used in clinical trials for medical research, biological studies, pharmaceutical trials, and now to test business ideas.
For the very first time in many business domains, experimentation reveals the causal impact of our business ideas. The results are humbling. They indicate that the vast majority of our business ideas fail to generate positive results. It’s not uncommon for 70-90% of ideas to either have no impact at all or actually move the metrics in the opposite direction of what was intended. Here are some statistics from a few notable companies that have disclosed their success rates publicly:
- Microsoft declared that roughly one-third of their ideas yield negative results, one-third yield no results, and one-third yield positive results (Kohavi and Thomke, 2017).
- Streaming service Netflix believes that 90% of its ideas are wrong (Moran, 2007).
- Google reported that as much as 96.1% of their ideas fail to generate positive results (Thomke, 2020).
- Travel site Booking.com shared that 9 out of 10 of their ideas fail to improve metrics (Thomke, 2020).
To be sure, the statistics cited above reflect a tiny subset of the ideas implemented by companies. Further, they probably reflect a particular class of ideas: those that are conducive to experimentation2 such as changes to user interfaces, new ad creatives, subtle messaging variants, and so on. Moreover, the companies represented are all relatively young and either in the tech sector or leverage technology as a medium for their business. This is far from a random sample of all companies and business ideas. So, while it’s possible that the high failure rates are specific to the types of companies and ideas that are convenient to test experimentally, it seems more plausible that the high failure rates are reflective of business ideas in general and that the disparity in perception of their success can be attributed to the method of measurement. We shouldn’t be surprised; high failure rates are common in many domains. Venture capitalists invest in many companies because most fail; similarly, most stock portfolio managers fail to outperform the S&P 500; in biology, most mutations are unsuccessful; and so on. The more surprising aspect of the low success rates for business ideas is most of us don’t seem to know about it.
(2) Most companies are unaware of the low success rates for their business ideas
Those statistics should be sobering to any organization. Collectively, business ideas represent the roadmap companies rely upon to hit their goals and objectives. However, the dismal failure rates appear to be known only to the few companies that regularly conduct experiments to scientifically measure the impact of their ideas. Most companies do not appear to employ such a practice and seem to have the impression that all or most of their ideas are or will be successful. Planners, strategists, and functional leaders rarely convey any doubts about their ideas. To the contrary, they set expectations on the predicted impact of their ideas and plan for them as if they are certain. They attach revenue goals and even their own bonuses to those predictions. But, how much do they really know about the outcomes of those ideas? If they don’t have an experimentation practice, they likely know very little about the impact their roadmap is actually having.
Without experimentation, companies either don’t measure the outcomes of their ideas at all or use flimsy methods to assess their impacts. In some situations, ideas are acted upon so fluidly that they are not recognized as something that merits measurement. For example, in some companies an idea such as “we’ll lower prices to increase demand by 10%” might be made on a whim by a marketing exec and there will be no follow up at all to see if it had the expected impact on demand. In other situations, a post-implementation assessment of a business idea is done, but in terms of execution, not impact (“Was it implemented on time?” “Did it meet requirements?” etc., not “What was the causal impact on business metrics?”). In other cases still, post hoc analysis is performed in an attempt to quantify the impact of the idea. But, this is often done using subjective or less-than-rigorous methods to justify the idea as a success. That is, the team responsible for doing the analysis often is motivated either implicitly or explicitly to find evidence of success. Bonuses are often tied to the outcomes of business ideas. Or, perhaps the VP whose idea it was is the one commissioning the analysis. In either case, there is a strong motivation to find success. For example, a company may seek qualitative customer feedback on the new loyalty program in order to craft a narrative for how it is received. Yet, the customers willing to give feedback are often biased towards the positive. Even if more objective feedback were to be acquired it would still not be a measure of impact; customers often behave differently from the sentiments they express. In still other cases, empirical analysis is performed on transaction data in an attempt to quantify the impact. But, without experimentation, at best, such analysis can only capture correlation—not causation. Business metrics are influenced simultaneously by many factors, including random fluctuations. Without properly controlling for these factors, it can be tempting to attribute any uptick in metrics as a result of the new business idea. The combination of malleable measurements and strong incentives to show success likely explain why so many business initiatives are perceived to be successful.
By contrast, the results of experimentation are numeric and austere. They do not care about the hard work that went into executing on a business initiative. They are unswayed by well-crafted narratives, emotional reviews by customers, or an executive’s influence. In short, they are brutally honest and often hard-to-accept.3 Without experimentation, companies don’t learn the sobering truth about their high failure rate. While ignorance is bliss, it is not an effective way to run your business.
(3) It is unlikely that companies will increase the success rate for their business ideas.
At this point, you may be thinking, “we need to get better at separating the wheat from the chaff, so that we only allocate resources to the good ideas.” Sadly, without experimentation, we see little reason for optimism as there are forces that will actively work against your efforts.
One force that is actively working against us is the way we reason about our companies.
We like to reason about our businesses as if they are simple, predictable systems. We build models of their component parts and manage them as if they are levers we can pull in order to predictably manage the business to a desired state. For example, a marketer seeking to increase demand builds a model that allows her to associate each possible price with a predicted level of demand. The scope of the model is intentionally narrow so that she can isolate the impact price has on demand. Other factors like consumer perception, the competitive assortment, operational capacity, the macroeconomic landscape, and so on are out of her control and assumed to remain constant. Equipped with such an intuitive model, she can identify the price that optimizes demand. She’s in control and hitting her goal is merely a matter of execution.
However, experimentation reveals that our predictions for the impact of new business ideas can be radically off—not just a little off in terms of magnitude, but often in the completely wrong direction. We lower prices and see demand go down. We launch a new loyalty program and it hurts retention. Such unintuitive results are far more common than you might think.
The problem is that many businesses behave as complex systems which cannot be understood by studying its components in isolation. Customers, competitors, partners, market force—each can adjust in response to the intervention in ways that are not observable from simple models of the components. Just as you can’t learn about an ant colony by studying the behaviors of an individual ant (Mauboussin, 2009), the insights derived from modeling individual components of a business in isolation often have little relevance to the way the business behaves as a whole.
It’s important to note that our use of the term complex does not just mean ‘not simple.’ Complexity is an entire area of research within Systems Theory. Complexity arises in systems with many interacting agents that react and adapt to one another and their environment. Examples of complex systems include weather systems, rain forest ecology, economies, the nervous system, cities, and yes, many businesses.
Reasoning about complex systems requires a different approach. Rather than focusing on component parts, attention needs to be directed at system-wide behaviors. These behaviors are often termed “emergent,” to indicate that they are very hard to anticipate. This frame orients us around learning, not executing. It encourages more trial and error with less attachment to the outcomes of a narrow set of ideas. As complexity researcher Scott E. Page says, “An actor in a complex system controls almost nothing but influences almost everything” (Page, 2009).
An example of an attempt to manage a complex system to change behaviors
To make this tangible let’s take a look at a real example. Consider the story of the child daycare company featured in the popular book, Freakonomics (the original paper can be found here). The company faced a challenge with late pickups. The daycare closed at 4:00pm, yet parents would frequently pick up their children several minutes later. This required staff to stay late causing both expense and inconvenience. Someone in the company had a business idea to address the situation: a fine for late pickups.
Many companies would simply implement the fine and not think to measure the outcome. Fortunately for the daycare, a group of researchers convinced them to run an experiment to measure the effectiveness of the policy. The daycare operates many locations which were randomly divided into test and control groups; the test sites would implement the late pickup fine while the control sites would leave things as is. The experiment ran its course and to everyone’s surprise they learned that fine actually increased the number of late pickups.
How is it possible that the business idea had the opposite effect of what was intended? There are several very plausible explanations, which we summarize below—some of these come from the paper while others are our own hypotheses.
The authors of the paper assert that imposing a fine makes the penalty for a late pick up explicitly clear. Parents are generally aware that late pick-ups are not condoned. But in the absence of a fine, they are unsure what the penalty may be. Some parents may have imagined a penalty much worse than the fine—e.g., expulsion from the daycare. This belief might have been an effective deterrent. But when the fine was imposed it explicitly quantified that amount of the penalty for the late pickups (roughly equivalent to $2.75 in 1998 dollars). For some parents this was a sigh of relief—expulsion was not on the docket. One merely has to pay a fine for the transgression, making the cost of a late pickup less than what was believed. Hence, late pick-ups increase (Gneezy & Rustichini, 2000).
Another explanation from the paper involves social norms. Many parents may have considered late pickups as socially inappropriate and would therefore go through great lengths to avoid them (leaving work early, scrambling for backup coverage, etc). The fine however, provides an easier way to stay in good social standing. It’s as if it signals ‘late pickups are not condoned. But if you pay us the fine you are forgiven.’ Therefore, the fine acts as the price to pay to stay in good standing. For some parents this price is low relative to the arduous and diligent planning required to prevent a late pickup. Hence, late pickups increase in the presence of the fine (Gneezy & Rustichini, 2000).
Still another explanation (which was only alluded to in the paper) has to do with the perceived cost structure associated with the staff having to stay late. From the parent’s perspective, the burden to the daycare of a late pickup might be considered fixed. If there is already at least one other parent also running late then there is no extra burden imposed since staff already has to stay. As surmised by the other explanations above, the fine increases the number of late pickups, which, therefore increases the probability that staff will have to stay late due to some other parent’s tardiness. Thus, one extra late pickup is no additional burden. Late pickups increase further.
One of our own explanations has to do with social norms thresholds. Each parent has a threshold for the appropriateness for late pickups based on social norms. The threshold might be the number of other parents observed or believed to be doing late pickups before such activity is believed to be appropriate. I.e., if others are doing it, it must be okay. (Note: this signal of appropriateness is independent from the perceived fixed cost structure mentioned above.) Since the fine increased the number of late pickups for some parents, other parents observed more late pickups and then followed suit.
The above are plausible explanations for the observed outcome. Some may even seem obvious in hindsight.4 However, these behaviors are extremely difficult to anticipate by focusing your attention on an individual component part: the fine. Such surprising outcomes are less rare than you might think. In this case, the increase in late pickups might have been so apparent that they could have been detected even without the experiment. However, the impact of many ideas often go undetected.
Another force that is actively working against our efforts to discern good ideas from bad is our cognitive biases. You might be thinking: “Thank goodness my company has processes that filter away bad ideas, so that we only invest in great ideas!” Unfortunately, all companies probably try hard to select only the best ideas, and yet we assert that they are not particularly successful at separating good from bad ideas. We suggest that this is because these processes are deeply human in nature, leaving them vulnerable to cognitive biases.
Cognitive biases are systematic errors in human thinking and decision making (Tversky & Kahneman, 1974). They result from the core thinking and decision making processes that we developed over our evolutionary history. Unfortunately, evolution adapted us to an environment with many differences from the modern world. This can lead to a habit of poor decision making. To illustrate: we know that a healthy bundle of kale is better for our bodies than a big juicy burger. Yet, we have an innate preference for the burger. Many of us will decide to eat the burger tonight. And tomorrow night. And again next week. We know we shouldn’t. But yet our society continues consuming too much meat, fat, and sugar. Obesity is now a major public health problem. Why are we doing this to ourselves? Why are we imbued with such a strong urge—a literal gut instinct—to repeatedly make decisions that have negative consequences for us? It’s because meat, fat, and sugar were scarce and precious resources for most of our evolutionary history. Consuming these resources at every opportunity was an adaptive behavior, and so humans evolved a strong desire to do so. Unfortunately, we remain imbued with this desire despite the modern world’s abundance of burger joints.
Cognitive biases are predictable and pervasive. We fall prey to them despite believing that we are rational and objective thinkers. Business leaders (ourselves included) are not immune. These biases compromise our ability to filter out bad business ideas. They can also make us feel extremely confident as we make a bad business decision. See the following sidebar for examples of cognitive biases manifesting in business environments and producing bad decisions.
Cognitive bias examples
Group Think (Whyte, 1952) describes our tendency to converge towards shared opinions when we gather in groups. This emerges from a very human impulse to conform. Group cohesion was important in our evolutionary past. You might have observed this bias during a prioritization meeting: The group entered with disparate, weakly held opinions, but exited with a consensus opinion, which everyone felt confident about. As a hypothetical example: A meeting is called to discuss a disagreement between two departments. Members of the departments have differing but strong opinions, based on solid lines of reasoning and evidence. But once the meeting starts the attendees begin to self censor. Nobody wants to look difficult. One attendee recognizes a gaping flaw in the “other side’s” analysis, but they don’t want to make their key cross functional partner look bad in front of their boss. Another attendee may have thought the idea was too risky, but, because the responsibility for the idea is now diffused across everyone in the meeting, won’t be her fault if the project fails and so she acquiesces. Finally, a highly admired senior executive speaks up and everyone converges towards this position (in business lingo we just heard the HiPPO or Highest Paid Person’s Opinion; or in the scientific vernacular, the Authority Bias (Milgram, 1963). These social pressures will have collectively stifled the meaningful debate that could have filtered out a bad business decision.
The Sunk Cost bias (Arkes & Blumer, 1985) describes our tendency to justify new investments via past expenditures. In colloquial terms, it’s our tendency to throw good money after bad. We suspect you’ve seen this bias more than a few times in the workplace. As another hypothetical example: A manager is deciding what their team will prioritize over the next fiscal year. They naturally think about incremental improvements that they could make to their team’s core product. This product is based on a compelling idea, however, it hasn’t yet delivered the impact that everyone expected. But, the manager has spent so much time and effort building organizational momentum behind the product. The manager gave presentations about it to senior leadership and painstakingly cultivated a sense of excitement about it with their cross functional partners. As a result, the manager decides to prioritize incremental work on the existing product, without properly investigating a new idea that would have yielded much more impact. In this case, the manager’s decision was driven by thinking about the sunk costs associated with the existing system. This created a barrier to innovation and yielded a bad business decision.
The Confirmation Bias (Nickerson, 1998) describes our tendency to focus upon evidence that confirms our beliefs, while discounting evidence that challenges our beliefs. We’ve certainly fallen prey to this bias in our personal and professional lives. As a hypothetical example: An exec wonders ‘should we implement a loyalty program to improve client retention?’ They find a team member who thinks this sounds like a good idea. So the exec asks the team member to do some market research to inform whether the company should create their own loyalty program. The team member looks for examples of highly successful loyalty programs from other companies. Why look for examples of bad programs? This company has no intention of implementing a bad loyalty program. Also, the team member wants to impress the exec by describing all the opportunities that could be unlocked with this program. They want to demonstrate their abilities as a strategic thinker. They might even get to lead the implementation of the program, which could be great for their career. As a result, the team member builds a presentation that emphasizes positive examples and opportunities, while discounting negative examples and risks. This presentation leads the exec to overestimate the probability that this initiative will improve client retention, and thus fail to filter out a bad business decision.
The biases we’ve listed above are just a sample of the extensive and well documented set of cognitive biases (e.g., Availability Bias, Survivorship Bias, Dunning-Kruger effect, etc.) that limit business leaders’ ability to identify and implement only successful business initiatives. Awareness of these biases can decrease our probability of committing them. However, awareness isn’t a silver bullet. We have a desk mat in our office that lists many of these cognitive biases. We regret to report that we often return to our desks, stare down at the mat … and realize that we’ve just fallen prey to another bias.
A final force that is actively working against efforts to discern good ideas from bad is your business maturing. A thought experiment: Suppose a local high school coach told NBA superstar Stephen Curry how to adjust his jump shot. Would implementing these changes improve or hurt his performance? It is hard to imagine it would help. Now, suppose the coach gave this advice to a local 6th grader. It seems likely that it would help the kid’s game.
Now, imagine a consultant telling Google how to improve their search algorithm versus advising a startup on setting up a database. It’s easier to imagine the consultant helping the startup. Why? Well, Google search is a cutting edge system that has received extensive attention from numerous world class experts—kind of like Steph Curry. It’s going to be hard to offer a new great idea. In contrast, the startup will benefit from getting pointed in a variety of good directions—kind of like a 6th grader.
To use a more analytic framework, imagine a hill which represents a company’s objective function5 like profit, revenue, or retention. The company’s goal is to climb to the peak, where it’s objective is maximized. However, the company can’t see very far in this landscape. It doesn’t know where the peak is. It can only assess (if it’s careful and uses experimentation) whether it’s going up or downhill by taking small steps in different directions—perhaps by tweaking it’s pricing strategy and measuring if revenue goes up.
When a company (or basketball player) is young, its position on this objective function (profit, etc.) landscape is low. It can step in many directions and go uphill. Through this process, a company can grow (walk up Mount Revenue). However, as it climbs the mountain, a smaller proportion of the possible directions to step will lead uphill. At the summit a step in any direction will take you downhill.
This is admittedly a simple model of a business (and we already discussed the follies of using simple models). However, all companies will eventually face the truism that as they improve, there are fewer ways to continue to improve (the low apples have been plucked), as well as the extrinsic constraints of market saturation, commoditization, etc. that make it harder to improve your business as it matures.6
So, what to do
We’ve argued that most business ideas fail to deliver on their promised goals. We’ve also explained that there are systematic reasons that make it unlikely that companies will get better, just by trying harder. So where does this leave you? Are you destined to implement mostly bad ideas? Here are a few recommendations that might help:
- Run experiments and exercise your optionality. Recognize that your business may be a complex system, making it very difficult to predict how it will respond to your business ideas. Instead of rolling out your new business ideas to all customers, try them on a sample of customers as an experiment. This will show you the impact your idea has on the company. You can then make an informed decision about whether or not to roll out your idea. If your idea has a positive impact, great. Roll it out to all customers. But in the more likely event that your idea does not have the positive impact you were hoping for you can end the experiment and kill the idea. It may seem wasteful to use company resources to implement a business idea only to later kill it, but this is better than unknowingly providing on-going support to an idea that is doing nothing or actually hurting your metrics—which is what happens most of the time.
- Recognize your cognitive biases, collect a priori predictions, and celebrate learnings. Your company’s ability to filter out bad business ideas will be limited by your team member’s cognitive biases. You can start building a culture that appreciates this fact by sending a survey to all of a project’s stakeholders before your next big release. Ask everyone to predict how the metrics will move. Make an anonymized version of these predictions and accuracy available for employees. We expect your team members will become less confident in their predictions over time. This process may also reveal that big wins tend to emerge from a string of experiments, rather than a single stroke of inspiration. So celebrate all of the necessary stepping stones on the way to a big win.
- Recognize that it’s going to get harder to find successful ideas, so try more things, and get more skeptical. As your company matures, it may get harder to find ways to improve it. We see three ways to address this challenge. First, try more ideas. It will be hard to increase the success rate of your ideas, so try more ideas. Consider building a leverageable and reusable experimentation platform to increase your bandwidth. Follow the lead of the venture world: fund a lot of ideas to get a few big wins.7 Second, as your company matures, you might want to adjust the amount of evidence that is required before you roll out a change—a more mature company should require a higher degree of statistical certainty before inferring that a new feature has improved metrics. In experimental lingo, you might want to adjust the “p-value thresholds” that you use to assess an experiment. Or to use our metaphor, a 6th grader should probably just listen whenever a coach tells them to adjust their jump shot, but Steph Curry should require a lot of evidence before he adjusts his.
This may be a hard message to accept. It’s easier to assume that all of our ideas are having the positive impact that we intended. It’s more inspiring to believe that successful ideas and companies are the result of brilliance rather than trial and error. But, consider the deference we give to mother nature. She is able to produce such exquisite creatures—the giraffe, the mighty oak tree, even us humans—each so perfectly adapted to their environment that we see them as the rightful owners of their respective niches. Yet, mother nature achieves this not through grandiose ideas, but through trial and error… with a success rate far more dismal than that of our business ideas. It’s an effective strategy if we can convince our egos to embrace it.
References
Arkes, H. R., & Blumer, C. (1985), The psychology of sunk costs. Organizational Behavior and Human Decision Processes, 35, 124-140.
Gneezy, U., & Rustichini, A. (2000). A Fine is a Price. The Journal of Legal Studies, 29(1), 1-17. doi:10.1086/468061
Kahneman, D., & Klein, G. (2009). Conditions for intuitive expertise: A failure to disagree. American Psychologist, 64(6), 515–526. https://doi.org/10.1037/a0016755
Kohavi, R. & Thomke, S. “The Surprising Power of Online Experiments,” Harvard Business Review 95, no. 5 (September-October 2017)
Mauboussin, M. J. (2009). Think Twice: Harnessing the Power of Counterintuition. Harvard Business Review Press.
Milgram, S. (1963). “Behavioral Study of obedience”. The Journal of Abnormal and Social Psychology. 67 (4): 371–378.
Moran, M. Do It Wrong Quickly: How the Web Changes the Old Marketing Rules . s.l. : IBM Press, 2007. 0132255960.
Nickerson, R. S. (1998), “Confirmation bias: A ubiquitous phenomenon in many guises”, Review of General Psychology, 2 (2): 175–220.
Page, S. E. (2009). Understanding Complexity – The Great Courses – Lecture Transcript and Course Guidebook (1st ed.). The Teaching Company.
Thomke, S. H. (2020). Experimentation Works: The Surprising Power of Business Experiments. Harvard Business Review Press.
Tversky, A., & Kahneman, D. (1974). Judgment under uncertainty: Heuristics and biases. Science, 185(4157), 1124-1131.
Whyte, W. H., (1952). “Groupthink”. Fortune, 114-117, 142, 146.
Footnotes
- Do not confuse the term ‘test’ to mean a process by which a nascent idea is vetted to get feedback. In an experiment, the test group receives a full-featured implementation of an idea. The goal of the experiment is to measure impact—not get feedback.
- In some cases there may be insufficient sample size, ethical concerns, lack of a suitable control group, and many other conditions that can inhibit experimentation.
- Even trained statisticians can fall victim to pressures to cajole the data. “P-hacking”, “significance chasing” and other terms refer to the temptation to use flawed methods in statistical analysis.
- We believe that these types of factors are only obvious in hindsight because the signals are often unobserved until we know to look for them (Kahneman & Klein, 2009).
- One reason among many why this mental picture is oversimplified is that it implicitly takes business conditions and the world at large to be static—the company “state vector” that maximizes the objective function today is the same as what maximizes the objective function tomorrow. In other words, it ignores that, in reality, the hill is changing shape under our feet as we try to climb it. Still, it’s a useful toy model.
- Finding a new market (jumping to a new “hill” in the “Mount Revenue” metaphor), as recommended in the next section, is one way to continue improving business metrics even as your company matures.
- VCs are able to learn about the outcomes of the startups even without experimentation. This is because the outcomes are far more readily apparent than that of business ideas. It’s difficult to cajole results to show a successful outcome when the company is out of business.